Protein design with deep learning
Peptides, such as human hormones, are important biomarkers in clinical care, e.g. levels of hormone PTH are measured to treat calcium imbalance or parathyroid disorders.
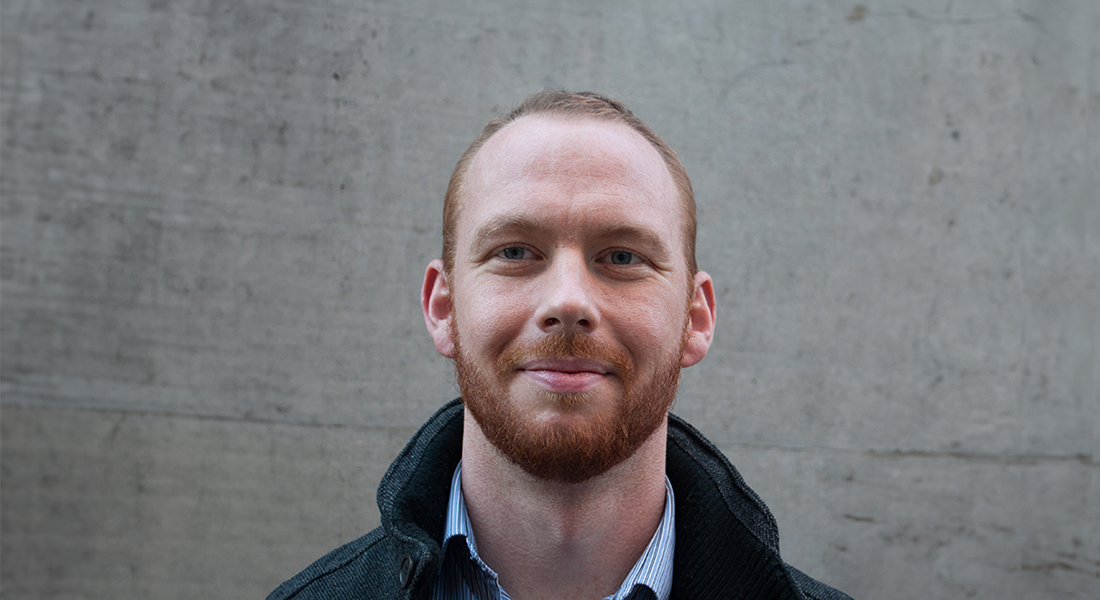
Proteins that can bind such short peptides can be used as diagnostic tools, or act as future therapeutics. In a collaboration with the David Baker lab, University of Washington, Associate Professor Joseph Matthew Rogers used deep learning methods to design new and stable proteins with a high capacity for peptide binding.
The research was published at the end of last year in the article “De novo design of high-affinity binders of bioactive helical peptides” in Nature.
Most of us have experimented for fun with the text-generating ChatGPT, one of the many advances in deep learning that have been made available recently. Advances similar to ChatGPT are occurring in biology, most notably with protein structure predictors such as AlphaFold and RoseTTAFold. These are well publicized, but what is less well known is that these structure predictors are also powerful tools for protein design - the generation of new proteins not seen before in nature. This research is an example of how biomedically useful proteins can be generated thanks to this deep learning revolution in biology.
Joseph Matthew Rogers describes the project:
“Proteins able to bind flexible, disordered peptides are abundant in nature, but efforts to design such proteins from scratch have been slow and limited to only particular types of flexible peptide. These deep learning methods are much faster, and promise to be more generally applicable to a greater range of peptide targets.
These new peptide-binding proteins could be used as sensors for peptide hormones, to aid diagnosis and therapeutic management of disease, or as powerful basic research tools to study and understand these diseases.
Teaming up with the protein design experts at the Baker lab, we ran the structure prediction tool AlphaFold in reverse to ‘hallucinate’ new proteins. Here, we used this hallucination approach to generate new protein sequences able induce folding of a pre-chosen, otherwise flexible, peptide. Experimentally, these designs were impressive: the proteins were highly stable and bound their peptides extremely tightly. The pace of developments in this area has been very fast, in this paper the Baker lab go on to demonstrate a range of other deep learning and non-deep learning methods to tackle the same problem.”
This paper includes the first example, to our knowledge, of ‘sequence-only’ design. Where only the amino acid sequence of the chosen peptide target is required to initiate the design of a binding molecule.”